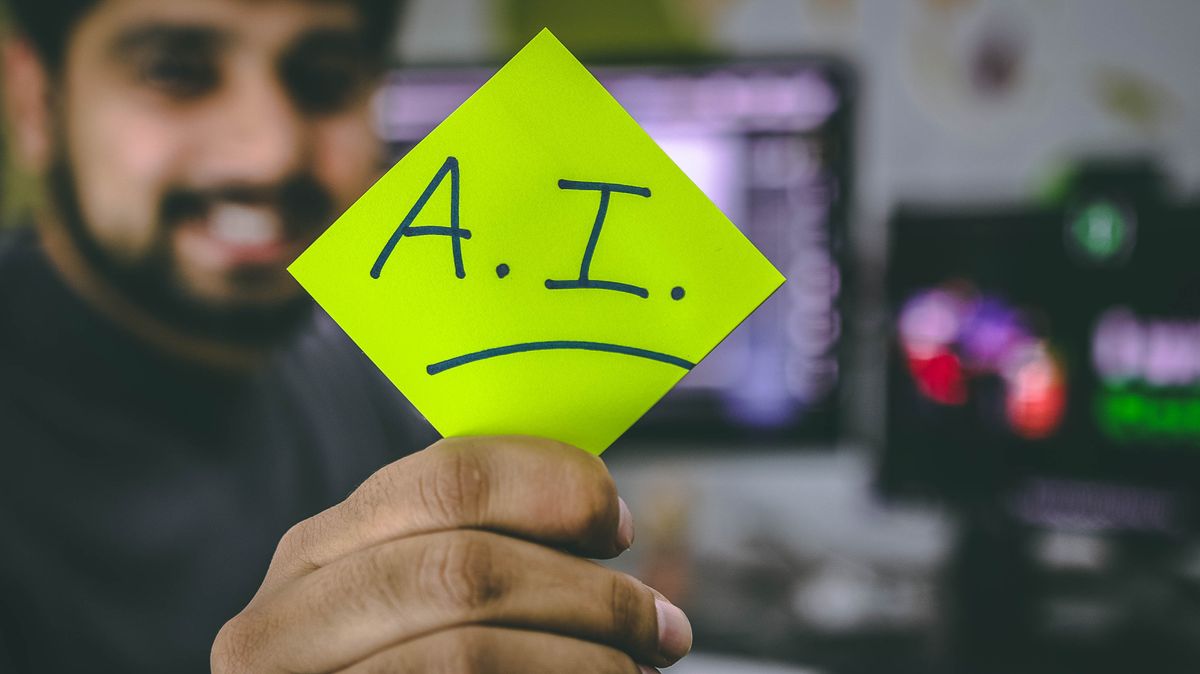
However, recent research suggests that XAI does not always achieve this goal (Branley-Bell, Whitworth & Coventry, 2020).
Discrepancies between developers’ and users’ interpretation can result in ineffective XAI.
This study uses an online experiment with human users to explore how user demographics (age, gender, domain experience) and type of XAI technique influence explainability.
Explainabillity is measured by three key desiderata: Understanding, uncertainty awareness and trust calibration.
The results indicate that different AI explanations can significantly impact users trust, understanding and ability to accurately predict uncertainty. Individual differences also play a significant role.
Based on the results, ‘XYZ’ algorithm appears to be most effective in relation to appropriate user trust, increased user understanding and more accurate awareness of uncertainty. Further experimental research is required. Significant effects of individual differences also suggest that it may be necessary to tailor XAI explanations to the specific user.
Partner: Red Hat
People: Dawn Branley-Bell